Machine learning meets decision making in agriculture
Purpose of cultivation advice 2.0
Farm21 is developing a self-learning cultivation decision supporting system. This is a platform in which agricultural data sources are combined and analysed by machine learning algorithms. The outcomes are evaluated and improved by user feedback. We call this Cultivation Advice 2.0. In this other blog we explain what we mean with this.
In this blog, however, we focus on what aspects are needed for setting up this system. We define the key elements and the starting points. These aspects are what we are working on day to day.
Cultivation advice 2.0 provides unique support for the individual grower. It enables the grower to make cultivation more efficient. This means: increasing yield, saving on valuable resources such as crop protection, fertilizer and water.
We believe that this is the future of digitalization in agriculture.
Machine learning as a flywheel
To save you from a very technical essay we explain machine learning on a very high level. Machine learning is an automated way of analysing large quantities of data. On a basic level, a well-functioning machine learning system consist of a continuous loop of the following steps.
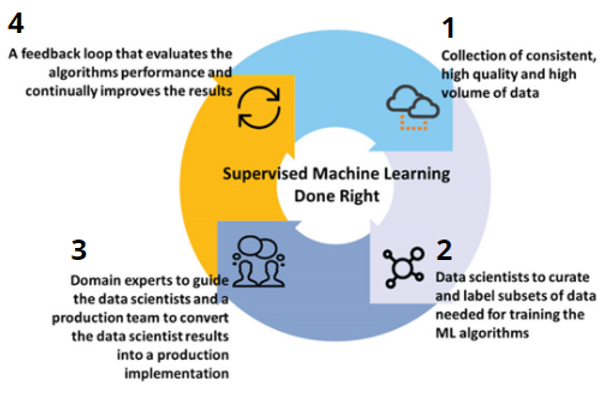
- Data collection. Consistent, high quality, high-volume data collection. An ever-growing dataset with as much (relevant) data as possible is the basis of the flywheel.
- Categorizing data. Data specialists categorize the data and train the ML algorithms. In our case, we identify a topic we want to analyse, say for instance a certain disease in a crop, and which parameters could potentially influence this outcome.
- Showing results. The results of the ML algorithms are shown to growers and crop consultants.
- Feedback. Feedback is processed and included in subsequent analyses.
To realize Farm21’s vision of the future and set the machine learning flywheel in motion, a number of key phases must be completed. This timeline shows the most important phases.
1 Collect data
Machine learning requires the consistent collection of high quality, high-volume data.
To achieve the right data density, it is necessary to reduce the cost per measurement point and to be able to link with already existing sensors.
To this end, we are developing proprietary sensors that enable fine-grained data collection as well as use existing sensors to build a data set of fine grit data.
2 Enrich data
By linking data sources together, it is possible for a machine learning algorithm to find correlations within the data.
Data sources we connect are for instance satellite imagery, crop databases, yield registration, soil scans, tractor data sets, and many more.
With information from different sources, it is also possible to filter out outliers. Improving the quality of the data used for training the machine learning algorithms.
3 Generic alerts
A pre-existing model (Generic Decision Support System) forms the basis of the alerts. Basic algorithms like “with temperature X, humidity Y over Z period of time, you have a high disease pressure.” These generic alerts are our starting point.
We collect when they are triggered and at a later stage relate these events to the response of the user.
4 Feedback
Feedback is the engine of the self-learning system. A simple “this is right/not right” already says a lot about the quality of advice. As mentioned before, we store this feedback and compare this to the given advice.
This way machine learning algorithms can determine on an individual level what the weighting factors should be for personal cultivation advice, but also use it to find more links between the datasets.
5 Predicting the future
Using the ever-growing data set, and the help of machine learning we can give advice or warnings about what might be coming.
The grower is thus informed earlier of climatic developments and their effect on his field and crop. This enables him to act more quickly.
6 Personalized crop advice
At this stage we reach crop advice 2.0 for the individual grower.
Fine-grained measuring allows cultivation actions to be carried out at a very precise level. Due to all the information that is fed into the system and analyses by machine learning algorithms, the level of insights is vastly increased. This results in localized, personalized farming advice.
Automated tailor-made cultivation advice is extremely detailed, works preventive but is targeted. It gives the chance to perform each action at the right time to optimize efficiency in yield and use of resources.